The Role of Human-Centric AI in Biotech Laboratories
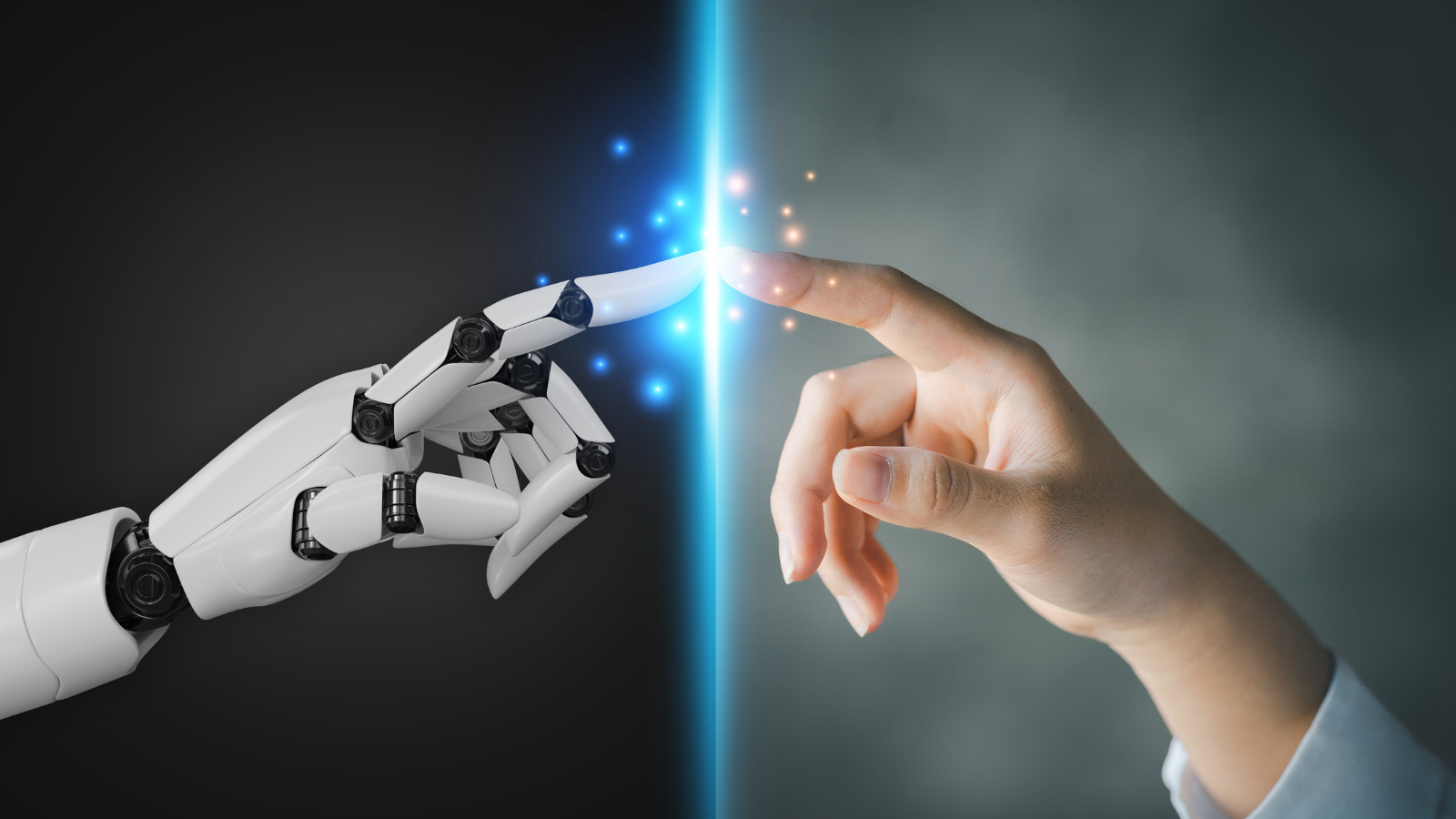
Download Whitepaper
In and out of the life sciences, the conversation about artificial intelligence (AI) is impossible to avoid. Because AI has crossed over into mainstream culture, the discussion about the pros and cons of its use is amplified. As with any new technology, there is fear and calls for an immediate half-year moratorium on research.
The flip side is the radical optimism espoused by Sam Altman of Open AI, with statements about its ability to improve the human condition. This sentiment is at the centre of the concept of human-centric AI, which we at eLabNext believe can benefit the biotech community. The following blog will discuss the basics of human-centric AI and how it can drive positive change in today’s modern biotech labs.
What is Human-Centric AI?
Human-centric AI refers to designing, developing, and deploying AI systems that prioritize the well-being, needs, and values of humans. In other words, it's the use of AI to improve the human condition.
Key Principles of Human-Centric AI
To ensure that AI systems are developed and deployed in ways that align with human interests, some guiding principles have emerged to help those actively engaged in AI work towards improving the human condition.
- Transparency & Explainability: Make AI systems explainable and understandable to humans, ensuring transparency in decision-making.
- Fairness and Avoiding Bias: Mitigate biases and guarantee fair treatment of individuals from diverse backgrounds, considering factors such as gender, race, or socioeconomic status.
- Privacy and Data Protection: Respect individuals' privacy rights and implement robust data protection measures to safeguard sensitive information.
- User Empowerment, User Control, and Autonomy: Design AI systems that empower individuals by giving them control, autonomy, and the ability to understand and influence AI's behaviour.
- Collaborative Interaction: Encourage human-AI collaboration and create AI systems that complement human capabilities, fostering teamwork and shared decision-making.
- Social & Environmental Impact: Assess the broader societal consequences of AI deployment and strive to address potential negative impacts while maximizing positive outcomes.
- Robustness and Reliability: Develop AI systems focusing on reliability, robustness, and safety, minimizing the potential for errors, biases, or unintended consequences. Adequate testing, validation, and risk assessment procedures should be in place.
- Ethical Governance: Integrate ethical considerations into all stages of AI development, including data collection, algorithm design, deployment, and monitoring.
Applying Human-Centric AI in Biotech
AI is already being applied in healthcare, where it’s being used to directly enhance the human condition with better disease detection and prediction.
Further upstream, in the biotech discovery or drug and diagnostic development spaces, human-centric AI enables vetting drug candidates, developing fruitful pre-clinical testing strategies, and more. There have been early adopters of AI systems and those who are more cautious, waiting until the dust clears to implement AI into their workflows.
Whether you fall into one camp or another, AI implementation in a laboratory environment requires a robust digital infrastructure. For those utilizing old-school, in-house built systems or pen-and-paper record-keeping with no long-term digitalization strategy, harnessing AI's power is bound to be a multi-stage, lengthy, and costly process. The foundation for being a human-centric AI biotech company is having a robust digital foundation across the board, from day-to-day sample management to large-scale raw file data control.
Ultimately, it comes down to having a Digital Lab Strategy that can lead your organization to implement human-centric AI more seamlessly, either now or in the not-too-distant future.
Start Your Lab’s Digital Journey Today
Are your samples and experiments digitized? Can you easily access and analyze your data? Is there a healthy collaborative culture and technical capability?
If so, then the rest is easy. Schedule a free personal demo with our digitalization specialist to get started!
Read more of our blogs about modern lab management
Discover the latest in lab operations, from sample management to AI innovations, designed to enhance efficiency and drive scientific breakthroughs.
Ready to transform your lab?
Enhance lab operations, improve collaboration, and ensure data security with eLabNext.
Experiment management
Inventory management
Improved collaboration
Protocol management
Research workflow management
.jpg)